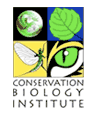
CBI investigated the effects of climate and vegetation on the distribution of martens (Martes caurina) and fishers (Martes pennanti) in the Sierra Nevada in California under current and projected future conditions to inform conservation efforts for these species and to investigate how different modeling methods and resolutions may affect predictions about species’ responses to climate change. Martens and fishers are closely related forest carnivores of conservation concern in California, where both reach their southernmost distributions. The species have contiguous elevation ranges, with the smaller marten occupying high subalpine forests that experience deep and persistent snow, and the larger fisher occupying mid-elevation forests that experience less snow and warmer temperatures.
The goals of this project were to:
- add robust, downscaled, climate-change effects assessments to CBI’S Sierra Nevada Carnivores project, which is a comprehensive, science-based effort to map areas important to sustaining rare carnivore populations and improving forest management.
- compare alternative analytical approaches and resolutions for assessing climate impacts on vegetation and sensitive species.
Because martens and fishers require similar forest structural conditions (dense forests with large trees and abundant dead wood) but different climate regimes (cooler, moister, and snowier conditions for martens; warmer, drier and less snowy conditions for fishers) they offer a unique opportunity to investigate how our changing climate may affect the species directly as well as via changes in vegetation. Also, because they compete with one another for food, and fishers will kill martens when they meet, this system offers an opportunity to investigate how species interactions may also affect future populations.
Specific study tasks:
- Examine how the current distributions of martens and fishers are influenced by vegetation characteristics (e.g., forest composition and structure), climate (e.g., temperature, precipitation, snow depth and duration), physical variables (e.g., elevation, % slope) and presence or absence of the other species.
- Project the potential future distribution of both species under climate change based on results of Task 1 and using alternative emissions scenarios and general circulation models at different resolutions.
- Use the results to support conservation and forest management plans to ensure long-term sustainability of marten and fisher populations in the face of climate change and increasingly severe fire regimes.
Geographic Location
Sierra Nevada and Cascades Ranges, California
Principal Investigators
Wayne D. Spencer
Dominique Bachelet
Ecosystem Type
Terrestrial
Framework focus
We used marten and fisher locality data collected throughout the Sierra Nevada and Cascades, mostly from systematic surveys using baited camera or track stations, and a wide array of environmental data sets to create marten and fisher distribution models for current conditions using the species distribution modeling software, Maxent (Phillips et al. 2006; software download). In so doing, we systematically varied the spatial resolution of the variables, and the types of variables included in distribution models, to determine what factors, at what resolutions, best predict species’ distributions under current conditions. The results were then used as a foundation for projecting future distributions (mid and late twenty-first century) using similar variables output by the MC1 dynamic global vegetation model (Bachelet et al. 2001). Climate projections were provided for several emission scenarios from different general circulation models at three different spatial resolutions (10x10 km, 4x4 km, and 800x800 m).
Current Species Distributions
We created and statistically compared a wide array of potential distribution models for each species using Maxent species distribution modeling software. The goal of these statistical analyses was to determine the optimal spatial resolution for assessing species distributions (at what landscape scale do these species select where to live?) and what types of variables best explain their current distributions (are they selecting primarily based on local climatic conditions, vegetation characteristics, the presence or absence of the other species, or combinations of factors?). We also examined correlations among variables to form hypotheses about which factors may be most important, and to avoid including highly correlated variables within a predictive model (e.g., we might use total annual precipitation or total winter precipitation in a model, but not both).
Because martens are more adapted than fishers to deep and persistent snow (Krohn et al. 1997, 2004), and both species show seasonal shifts in habitat use (our results), we also created different distribution models for the predominantly snow-free period (summer = May to November), the snowy period (winter = December to April) and year-round for each species.
We created and compared a large array of potential distribution models using the following factors:
- 32 different environmental variables (10 climate variables, 16 vegetation composition and structure variables, 6 physical variables) plus presence or absence of the other Martes species, with 0.1-km cell resolution (100x100 m).
- Seven different spatial smoothing scales (averaging of environmental variables over moving window sizes ranging from 1 to 3000 ha).
- Variables and spatial smoothing scales yielding the strongest correlations with species’ distributions were combined into six different model "types", defined using variable categories:
- climate only.
- vegetation only.
- climate + vegetation.
- climate + vegetation + physical.
- climate + vegetation + presence/absence of the other Martes species.
- climate + vegetation + physical + presence/absence of the other Martes species.
After creating this array of potential predictive models, we compared them statistically to determine which factors, at which scales, most strongly predict species’ distributions year-round or during the summer or winter. We compared AUCs (area under curve; a measure of model fit that varies from 0 to 1.0) using 10-fold cross-validation, t-tests, and other metrics to determine the best predictive models and the relative contribution of different variables to model predictive strength. These statistical comparisons revealed how these species currently distribute themselves on the landscape in response to these different types of factors, so that we can better understand how they are likely to respond to future changes in climate and vegetation.
Future Projections
The preceding comprehensive analysis of current distribution models demonstrated that climate + vegetation models, at scales corresponding roughly with species’ home range sizes, performed best. Informed by these analyses, we next created climate + vegetation models using variables output by the MC1 global vegetation model, and which can be projected into the future. These distribution models used projections from five different climate models/emissions scenarios at various spatial resolutions, averaged over three, 20-year time periods:
- Scenarios and Resolutions:
- PCM A2 (10x10 km)
- HADLEY A1Fi (10x10 km)
- MIROC A2 (4x4 km and 800x800 m)
- HADLEY CM3 A2 (4x4 km and 800x800 m)
- CSIROMk3 A2 (800x800 m)
- Time Periods:
- Contemporary (1986- 2005)
- Mid century (2046-2065)
- Late century (2076-2095)
Results of the MC1-based distribution models for the contemporary time period were compared with the best models derived earlier for current conditions to determine whether they gave comparable results and were therefore reliable predictors of marten and fisher distributions. The best MC1-based models were then projected to the mid- and late-century time periods under each emission/global circulation/resolution scenario. Finally, we overlaid results of the various scenarios at each time period to assess degree of concordance among the various scenarios.
Current Species Distributions
The univariate resolutions that best predicted species’ distributions varied among the species and variables tested, but in general AUCs tended to be higher at fine to moderate spatial smoothing scales (1 to 20 km2) for fishers, and at moderate to coarse (10 to 30 km2) scales for martens. However, the influence of smoothing scale on predictive power was quite modest for most variables (most comparisons were not statistically significant). We therefore used 10-km2 smoothed variables for the multivariate model comparisons, because this moderate resolution performed well across most variables and both species, and it is biologically justified as approximating the habitat area needed to support one to a few individuals of each species.
The distributions of martens and fishers were well predicted by both vegetation-only or climate only models (all AUCs > 0.8), but models combining both vegetation and climate variables performed better than models based on either climate or vegetation alone for both year-round and seasonal distributions (the one exception being for martens during winter, when there was no significant difference between the vegetation + climate model and the climate-only or vegetation-only model) (Table 1). Climate is a strong predictor of habitat characteristics for these species, but cannot account for vegetation disturbance history or other factors that also influence habitat quality. Adding physical variables or the presence-absence of the other Martes species did not improve model predictive power relative to vegetation + climate models.
Table 1: AUC values for different model types for predicting contemporary distributions of martens and fishers during summer, winter, and year-round. Vegetation + climate models performed better than climate-only or vegetation-only models; adding physical variables or presence of the other Martes species did not improve predictions of vegetation + climate models.
|
Fisher |
Marten |
Model |
Summer |
Winter |
Year Round |
Summer |
Winter |
Year Round |
VEG |
0.802 |
0.871 |
0.809 |
0.845 |
0.837 |
0.836 |
CLIM |
0.841 |
0.848 |
0.824 |
0.852 |
0.847 |
0.837 |
VEG + CLIM |
0.885v,c |
0.933v,c |
0.871v,c |
0.871v,c |
0.856 |
0.858c |
VEG, CLIM, + PHYS |
0.884 |
0.944 |
|
|
|
|
VEG, CLIM, + MARTES |
0.885 |
0.993 |
0.865 |
0.874 |
0.856 |
0.858 |
VEG, CLIM, PHYS, + MARTES |
|
0.944 |
|
|
|
|
v Significantly better than vegetation only model (P < 0.05).
c Significantly better than climate-only model (P < 0.05).
Variables entering the top-performing models support previous findings that both martens and fishers select forest vegetation with dense canopies, large trees, and abundant coarse wood, but that martens are associated with higher-elevation forest types (red fir, lodgepole pine) whereas fishers are associated with mid-elevation forest types (Sierran mixed conifers, mixed conifer-hardwoods). Both species appear to select from a fairly narrow range of climate conditions compared to what is available in the region, with clear differences between the preferences of the two species. Martens are closely associated with areas having low summer temperatures, relatively stable daily temperatures (i.e., low amplitude between daily highs and lows), abundant precipitation, and deep snow that persist through March. Fishers are associated with somewhat higher temperatures than martens (but still having low daily temperature amplitudes), areas with lower annual and winter precipitation than martens, and areas with little snow remaining by March.
Once effects of both climate and vegetation are included in a model, adding presence or absence of the other Martes species did not contribute significantly to model predictive power. These results suggest that martens and fishers primarily select habitats based on appropriate climate and vegetation conditions, rather than the presence or absence of the other species. Presence of fishers may affect some individual martens in their narrow contact zone, but does not appear to be a driving factor behind the distribution of marten populations.
Both species showed significant seasonal patterns in distribution (Figure 1). During winter, fishers contract their ranges to a narrower, lower, elevation range than they occupy during summer, apparently to avoid deep snow. Martens, conversely, appear to expand their ranges downslope during winter, so that martens and fishers maintain essentially contiguous ranges year-round where they co-occur (south from Yosemite National Park). Presence or absence of the other species does not appear to be the mechanism behind these patterns, however, because martens expand and contract their habitat use seasonally even in regions lacking fishers (north of Yosemite National Park).
Figure 1: Martens (red) and fishers (blue) have contiguous ranges year round, with seasonal shifts: martens expand downslope and fishers contract downslope during winter.
Current distributions using MC1 models
Of three resolutions available for MC1 results, the intermediate 4x4 km resolution performed best at predicting the current distributions of martens and fishers (Table 2). This resolution is similar to the 10km2 (= 3.3x3.3 km) used in the previous contemporary analyses. The 10x10 km (= 100km2) models were too coarse in resolution and appear to grossly over-estimate species distributions compared with our best current-condition models (predicting 1.7 to 2.7 times as much area with probability of species occurrence > 0.5). Somewhat surprisingly, the finer resolution 800x800 m models did not improve predictions over 4x4 km models, perhaps because this is finer than the scale at which these species select habitats, as assessed above.
Table 2: AUC values for models predicting the contemporary distribution of martens and fishers based on variables derived from MC1 at different resolutions. The 4x4 km resolution performed best for all comparisons.
|
Fisher |
Marten |
Scenario |
Summer |
Winter |
Year Round |
Summer |
Winter |
Year Round |
10x10 km (HAD A1) |
0.813 |
0.872 |
0.819 |
0.803 |
0.794 |
0.785 |
10x10 km (PCM A2) |
0.814 |
0.884 |
0.818 |
0.795 |
0.791 |
0.780 |
4x4 km |
0.891*^ |
0.916 |
0.873*^+ |
0.860*^ |
0.877*^ |
0.863*^ |
800x800 m |
0.827 |
0.877 |
0.836 |
0.856 |
0.864 |
0.848 |
* Significantly better than 10x10 km PCM A2 (P < 0.05).
^ Significantly better than 10x10 km HAD A1 (P < 0.05).
+ Significantly better than 800x800 m (P < 0.05).
At the best resolution of 4x4 km, climate + vegetation models always significantly outperformed vegetation-only models (P < 0.005); climate + vegetation models also slightly outperformed climate-only models, but most differences were not significant (except for marten year-round, where P < 0.05). Model performance was very good for the 4x4 km vegetation + climate MC1-derived models (AUC values of 0.86 to 0.92, Table 2) and compare favorably with those from the previous analyses (0.86 to 0.93, Table 1), suggesting that these MC1-based models are reliable predictors of species’ distributions.
Future Projections
All scenarios paint a rather dire picture for the future of these species in the Sierra Nevada, with major contractions in predicted suitable habitat area (i.e., probability of occupancy > 0.5) (marten, fisher) coupled with greatly increased habitat fragmentation and generally upslope shifts in distribution. Under some scenarios, a major contraction in suitable habitat by the mid twenty-first century is followed by a slight expansion again by the end of the century, suggesting that there may be a mid-century bottleneck that populations must pass through. Because our models used both climate and vegetation variables, this contraction followed by slight expansion may represent a decoupling of the species’ preferred climatic vs. vegetation conditions, with shifts in vegetation communities lagging behind the shifts in climate.
There remain large uncertainties about future conditions, due to differences among the different scenarios used for projections. Climate models agree over the general warming trend in the western US, but projections of precipitation are much less certain, increasing the uncertainty in projections of future drought stress and fuels conditions and fire hazards. Projections of snowpack depth and duration are highly uncertain and also increase the uncertainty in simulating future habitat conditions. Increased climate variability may allow for some very high snow years as well as extremely low snow years; how marten and fisher populations may respond to this interannual variability is uncertain.
New climate projections from the 5th assessment report are coming available (e.g. Peacock 2011) and indicate further declines in spring snow cover and increases in summer-time temperatures. As more climate modeling teams provide their latest results, ensemble means will show whether updated model runs will bring new trends in climate conditions over the western US, but early results do not show any lessening of the already documented trends.
Decoupling of climate envelopes from vegetation conditions, due to vegetation lags, may spell trouble for species like martens and fishers whose niches are strongly influenced by both. However, these correlation analyses cannot attribute cause/effect relations, and it remains unknown whether these species will abandon current vegetation community associations to stay within preferred climate envelopes (so long as forest structural conditions are appropriate). The MC1 vegetation model simulates potential vegetation associated with climate conditions, with rapid shifts from one type of land cover to another, especially facilitated by simulated disturbance such as fire. However, fire suppression and long term legacy from existing forests may in reality buffer such rapid shifts and allow wildlife species more time to respond to change than models are projecting. Nonetheless, rapid shifts due to increased vulnerability of forests to pest outbreaks, and severe wildfires due to drought conditions and warming, have been observed recently in western mountains where widespread mortality has changed habitat conditions much faster than previously expected (Westerling et al. 2006, Wiedinmeyer et al. 2012).
Downscaling to ever finer-resolution GCMs does not necessarily improve species distribution predictions. Martens and fishers appear to select landscapes at resolutions of roughly 2-30 km2 so finer downscaling (e.g., 800x800 m) may not increase model accuracy. Also, complex topography can cause microclimate conditions that are not represented by interpolated climate from a limited number of meteorological stations, allowing some decoupling from regional climate (Daly et al. 2009). Complex terrain in the Sierra Nevada may cause inversions and cold air ponding, for example, which may result in refugia with appropriate climate and vegetation conditions that cannot be predicted with the current models. Furthermore, riparian corridors will provide cooler and moister conditions that can create at least temporary refugia for species affected by new climate conditions.
Based on the results of these analyses and our general knowledge of marten and fisher ecology, we suspect that martens may persist in areas that retain deep, persistent snow and some dense forest patches with large trees and woody structures, perhaps in high-elevation canyons and valleys, although their populations are likely to be much more fragmented in the future. Fishers may shift upslope into vegetation communities currently occupied by martens (e.g., red fir) as deep, persistent snow cover declines due to climate change, so long as these areas continue supporting some dense forests with large woody structures. However, there is great uncertainty about the ability of either or both species to adapt and persist in the face of climate change in the Sierra Nevada, given the high degree of habitat loss and fragmentation projected by our models.